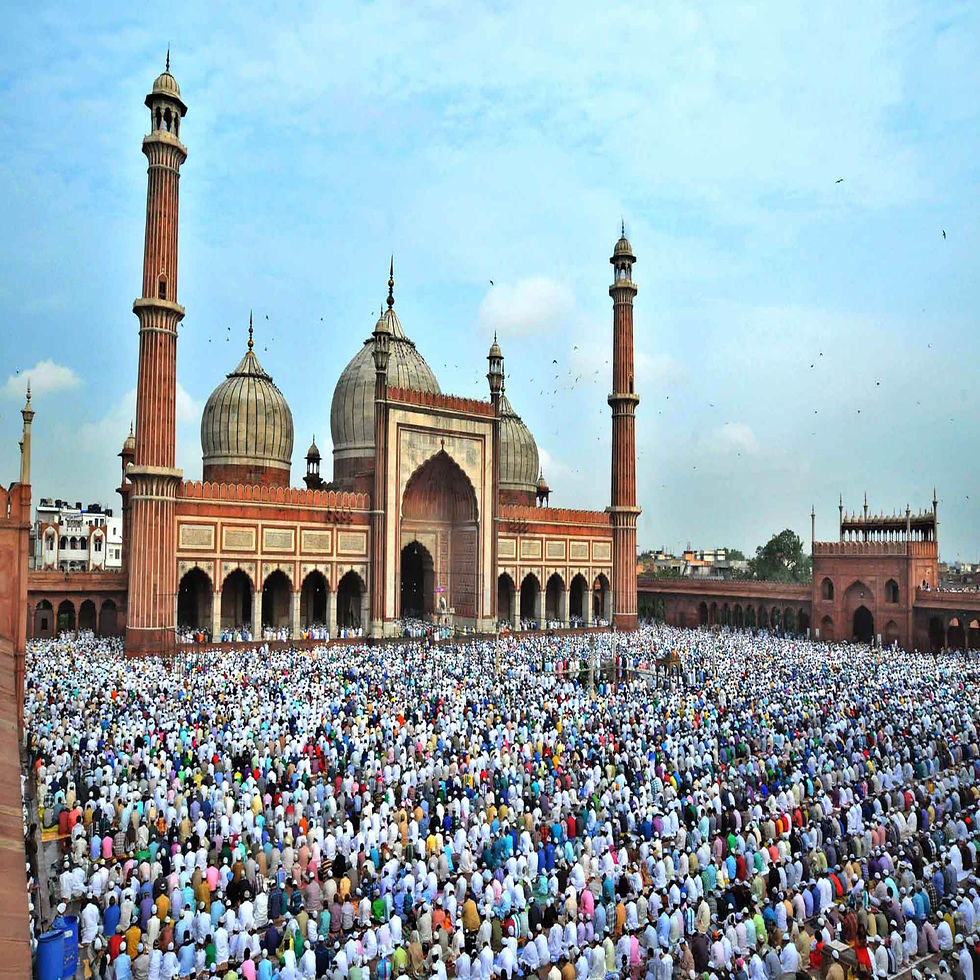
FastPval Crack Registration Code [Mac/Win] [Updated-2022] 1. FastPval Product Key can process very large datasets. Let's say the dataset size is 100GB. We will split the dataset into 20GB parts based on user's p-value (default: 10^-5). We will do that until p-value = 10^-5. The 20GB parts will be stored in memory. 2. Each partition has a preset mode to store data, for example: binary matrix. 3. The software will create a model based on the background data for each partition. 4. Each partition will be used to calculate p-value for background using different model. 5. Results are stored and can be compared with each other. 6. FastPval For Windows 10 Crack will be much faster than traditional method that relies on storing the whole dataset. 7. FastPval will be memory efficient. For example, if we have 100GB data, and the user specified p-value is 10^-5, then the data will be split into 20GB parts. The size of each part will be about 10GB. Using the default mode, FastPval will use 2GB memory only. 8. To use fastPval, all we need is to pass the file containing the dataset to the software and specify the p-value for each partition. Applications FastPval is currently used in the following software packages: 1. The R package "fastPval" 2. The Bioconductor package "twilight" 3. The R package "getopt" 4. The R package "pROC" Installation Firs install R with: > install.packages("r-release") Next, install R package "fastPval": > install.packages("fastPval") Configuration User can specify different parameters for FastPval. The default parameters are suitable for most cases. Parameters that we suggest users to consider: - p-value: user specify a p-value to do the analysis (default: 10^-5) - FastPval Crack + (April-2022) FastPval was developed by C Cairo, and C Texas A&M. It was developed in C++, and it's open source. The framework is built on the boost C++ library. FastPval Features: FastPval provides a generic way to separate a dataset into smaller sets, build models for each set, calculate p-value based on the models, and then output the p-value and the associated samples of the original dataset. FastPval Usage: To use FastPval you just need to specify the background file and the new file, the datasets contained in the background file are sorted in reverse order according to p-values. FastPval Usage: Input Parameters: { & 1a423ce670 FastPval Crack Torrent data= all_data.csv #inputs: #- 'n' : the size of the part in the dataset #- 'p_value_to_split' : the p-value to split the dataset #-'sample_size' : the size of the resampled part #-'max_m' : the model to construct #-'model_size' : the size of the model used #-'split_points' : the start index and the end index to separate the dataset #- 'comparison_method' : the comparison method to search the p-value #-'resampled_data' : the resampled data #output: #-'score' : the p-value from the resampled data #- 'part' : the p-value of the resampled part in the original dataset #-'model' : the logistic model used to calculate the p-value #-'resampled_data_list' : the p-values of the resampled data after sorted and search by score #- 'n' : the size of the part in the dataset data= all_data.csv #inputs: #- 'n' : the size of the part in the dataset #- 'p_value_to_split' : the p-value to split the dataset #-'sample_size' : the size of the resampled part #-'max_m' : the model to construct #-'model_size' : the size of the model used #-'split_points' : the start index and the end index to separate the dataset #- 'comparison_method' : the comparison method to search the p-value #-'resampled_data' : the resampled data #output: #-'score' : the p-value from the resampled data #- 'part' : the p-value of the resampled part in the original dataset #-'model' : the logistic model used to calculate the p-value #-'resampled_data_list' : the p-values of the resampled data after sorted and search by score #- 'n' : the size of the part in the dataset data= all_ What's New in the? System Requirements For FastPval: Windows 7, 8, 8.1, 10 Minimum: OS: Windows 7, 8, 8.1, 10 Processor: AMD A-Series, Intel 4th-Generation Core or AMD FX-Series, Intel i5-series or AMD Ryzen 3rd-Generation Memory: 6 GB RAM Graphics: AMD Radeon HD 5770 (HD 5870) or NVIDIA Geforce GTX 460 or better Storage: 5 GB available space Internet: Broadband Internet connection Additional Notes:
Related links:
Comments